Empowering Ultra-Precision Agriculture with Machine Learning, Modeling, and Robots
CyVerse is part of a multi-institutional effort to integrate diverse, progressive technologies to replace farmers' reliance on heavy machinery and pesticides.
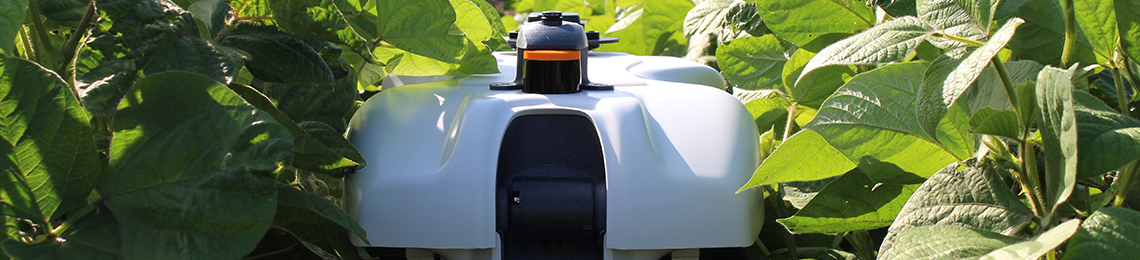
A robot developed by University of Illinois researchers in a soybean test plot at Iowa State University. Photo credit: Iowa State University.
A gardener hoping for a crop of the juiciest summer tomatoes might tend to each and every plant in a plot. But a farmer working to feed the world?
Researchers believe it's possible. They're applying and integrating layers of technologies – including sensors, machine learning, artificial intelligence, high-throughput phenotyping platforms such as drones and small-scale rolling robots that can also fertilize, weed and cull single plants in a field – with the ultimate goal of replacing farmers' reliance on heavy machinery and broadcast spraying.
The researchers call their effort COALESCE – COntext Aware LEarning for Sustainable CybEr-agricultural systems. They have just won a five-year, $7 million Cyber-Physical Systems Frontier award jointly funded by the National Science Foundation and the U.S. Department of Agriculture's National Institute of Food and Agriculture.
Introducing the latest cyber capabilities in sensing, modeling and reasoning to the real world of plants and soil, the researchers wrote in a project summary, will "enable farmers to respond to crop stressors with lower cost, greater agility, and significantly lower environmental impact than current practices."
The CyVerse team will contribute its robust computational infrastructure to democratize access to the machine learning and modeling tools and methods used, making it possible for other teams to reproduce and adapt the project's components.
To enable the large-scale, highly distributed data aggregation, quality control, and machine learning, CyVerse will adapt its cloud native platform and deploy edge cloud components for use in the field.
"By delivering machine learning to execute directly at the field as data is gathered from the sensors, rather than waiting for data to be streamed into a central location, researchers can receive notifications and make decisions much faster, almost immediately," said Edwin Skidmore, CyVerse's Director of Infrastructure.
"The leadership that we've developed over the years in cloud computing and cyberinfrastructure for managing large-scale datasets and complex workflows will be the foundation for this team," said Nirav Merchant, CyVerse co-principal investigator and Director of the University of Arizona's Data Science Institute.
The lead principal investigator for the project is Soumik Sarkar, the Walter W. Wilson Faculty Fellow in Engineering and an associate professor of mechanical engineering at Iowa State University. A partner principal investigator is Girish Chowdhary, an associate professor of agricultural and biological engineering at the University of Illinois Urbana-Champaign.
In addition to CyVerse at the University of Arizona, the research team also includes collaborators from George Mason University in Virginia, the Iowa Soybean Association, and Ohio State University.
Beyond precision agriculture
"You hear about precision agriculture all the time," Sarkar said, referring to the practice of monitoring crops and soils to make sure they get exactly what they need for optimal production, while also reducing the need for fertilizers, pesticides and other expensive and potentially polluting inputs. "Now, we're trying to move another notch above that."
Call that "ultra-precision agriculture, which is scale agnostic," said Asheesh (Danny) Singh, a professor of agronomy and the Bayer Chair in Soybean Breeding at Iowa State.
"A lot of agricultural problems start in a small area of a field," he said. "We want to localize problems early on – make decisions and start controls before they affect the whole field and adjoining farms. Working at the plant level gives us that ultra-high precision with row crops such as soybeans."
And, the researchers said, the technology would also be affordable and accessible enough to help producers who grow vegetables and other specialty crops on farms of various sizes.
Data-driven decisions
The ideas behind COALESCE have been bubbling around the Iowa State campus for years and have led to the creation of a core research team: Sarkar; Singh; Baskar Ganapathysubramanian, the Joseph C. and Elizabeth A. Anderlik Professor in Engineering; and Arti Singh, an assistant professor of agronomy.
The ideas have also attracted several competitive grants, including an initial grant to the core team from the Iowa Soybean Association with Arti Singh as the principal investigator. There was also a three-year seed grant to the core team from Iowa State's Presidential Initiative for Interdisciplinary Research. These grants helped build the team, make initial discoveries and connect with other researchers.
An illustration from the seed project – a project called "Data Driven Discoveries for Agricultural Innovation" – shows an airplane, three drones and four robots collecting data from a field to help the farmer standing to the side.
How can all that data help a farmer?
"Data science isn't just about assembling data and making predictions," Ganapathysubramanian said. "It's also about making decisions."
Where, for example, are plants stressed by pests, or dry conditions or poor soils? And what can be done about it?
Thanks to a partnership with the Iowa Soybean Association, those kinds of data-to-decision scenarios have been discussed with farmers.
And, said Arti Singh, farmers are interested in the promise of ultra-precision agriculture.
"They're the ones who said, 'yes, this is possible,'" she said.
But it will take work to get there.
Development of an ultra-precision, cyber-physical system for agriculture "cannot happen without the level of investment provided by this Frontier project," Asheesh Singh said. "And without the expertise on this team, and the partnership with farmers, work like this cannot happen."